Description
The MSU LFW+ database was created by extending the LFW database [1] to study the joint attribute learning/estimation (age, gender, and race) from unconstrained face images. Since the number of young subjects (e.g., in the age group 0-20) in the LFW database is very small (only 209 subjects among the 5,749 subjects according to the labels provided by MTurkworkers), the LFW database was extended by collecting 2,466 unconstrained face images of subjects in the age range 0-20 years using Google Images search service. Specifically, we first used the keywords such as “baby”, “kid”, and “teenager” to find about 5,000 images of interest from Google Images. The Viola-Jones [54] face detector was then applied to generate a set of candidate faces. Finally, we manually removed false face detections as well as most of the subjects that appeared to be older than 20. The extended LFW database (LFW+) contains 15,699 unconstrained face images of about 8,000 subjects. For each face image, three MTurk workers were asked to provide their estimates of age, gender, and race. The apparent age is determined as the average of the three estimates, and the gender and race are determined by the majority vote rule. A project page could be found here.
The database was created at Michigan State University’s Pattern Recognition and Image Processing (PRIP) Lab, in East Lansing, MI, US. Here are some example of the face images and their age, gender, and race labels.
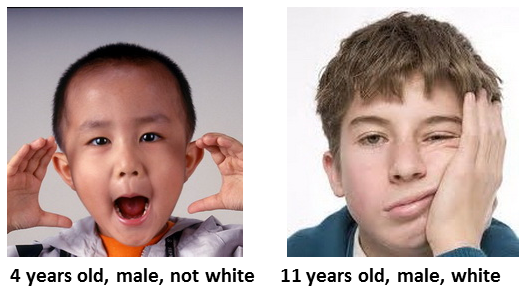