Description
The MSU USSA database was created to simulate spoof attacks on smartphones. With the release of Android 4.0, millions of smartphones now have the ability to unlock their device using the Trusted Face (face unlock) functionality such as the Google Nexus 5. Hence, the cameras used in capturing the MSU USSA database simulate the input the Trusted Face application would receive when a malicious user tries to spoof the system. Spoof attacks captured by DSLR or USB webcams do not replicate the application of user authentication on smartphones as they contain additionally sensors (DSLR Cameras) or capture blurry out of focus images (USB webcams).
Moreover, current public-domain spoof databases lack diversity in terms of background, illumination, and image quality, and thus do not replicate real application scenarios. The MSU USSA database was also created to ensure that it contains a mixture of environments, image qualities, image capture devices and subject diversity. To create such a database we selected 1,000 live subject images of celebrities from the Weakly Labeled Face Database (http://wlfdb.stevenhoi.com). Therefore, the public set of the MSU USSA database for face spoofing consist of 9,000 images (1,000 live subject and 8,000 spoof attack) of the 1,000 subjects.
Due to IP issues, the public set we released is slightly different from the public set used in our paper (the genuine and spoof images of the subjects from the Idiap and CASIA databases are not included). In order to allow other researchers to compare their results with our results, this webpage contains spoof detection performance on the new public set of the MSU USSA database. Please note there is only a slight change in the performance results.
The database was produced at Michigan State University’s Pattern Recognition and Image Processing (PRIP) Lab, in East Lansing, MI, US.
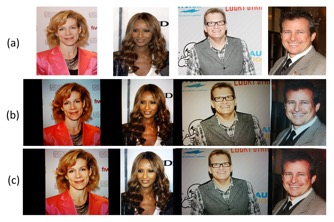
Figure 1: Sample images of (a) live faces, (b) spoof faces captured by the front facing camera, (c) spoof faces captured by rear facing camera on the Nexus 5. The 4 spoof images are captured using 4 spoof mediums in the following order MacBook, Nexus 5, Nvidia Shield Tablet, and Printed Photo.
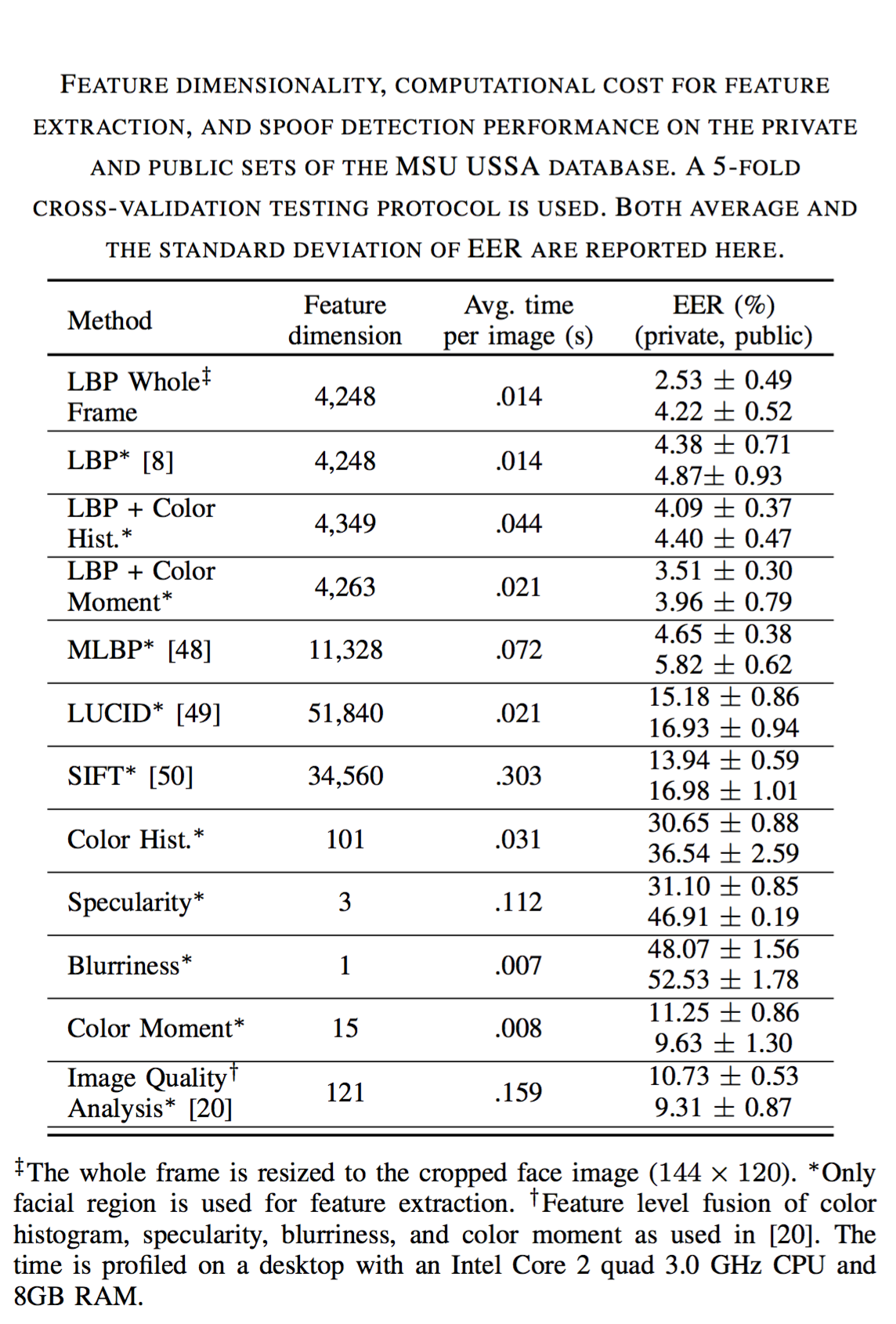