 |
Current Projects
Demographic Attribute Estimation from Face Images
Demographic estimation entails automatic estimation of age, gender and race of a person from his face image, which has many potential applications ranging from forensics to social media.Automatic demographic estimation, particularly age estimation, remains a challenging problem because persons belonging to the same demographic group can be vastly different in their facial appearances due to intrinsic and extrinsic factors. In this work, we present a generic framework (see Fig. 2) for automatic demographic (age, gender and race) estimation. Given a face image, we first extract demographic informative features via a boosting algorithm; we then employ a hierarchical approach consisting of between-group classification and within-group regression. Quality assessment is also developed to identify low-quality face images from which reliable demographic estimates are difficult to obtain. Experimental results on a diverse set of face image databases, FG-NET (1K images), FERET (3K images), MORPH II (75K images), PCSO (100K images), and a subset of LFW (4K images), show that the proposed approach has superior performance compared to the state of the art. We additionally use crowdsourcing to study the ability of human perception to estimate demographics from face images. A side-by-side comparison of the demographic estimates from crowdsourced data and the proposed algorithm provides a number of insights into this challenging problem.
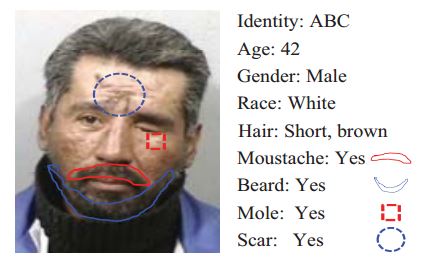 |
Fig. 1. A wide variety of information can be gleaned from a face image, such as identity, age, gender, race, and scars, marks and tattoos (SMT). |
 |
Fig. 2. Overview of the proposed approach for automatic demographic estimation. I and Γ are the input and preprocessed face images, respectively; ψ and Ψ denote the BIF extraction process, and D → S denotes the demographic informative feature selection; x and x' denote the BIF feature vector before and after feature selection, respectively; and QA(⋅) is the quality assessment process. |
Relevant Publication(s)
1. H. Han, C. Otto, X. Liu, and A. K. Jain. Demographic Estimation from Face Images: Human vs. Machine Performance. IEEE Transactions on Pattern Analysis and Machine Intelligence, 2015 (To Appear). [pdf]
2. H. Han, C. Otto, and A. K. Jain. Age Estimation from Face Images: Human vs. Machine Performance. In Proc. 6th IAPR International Conference on Biometrics (ICB), Madrid, Spain, June 4-7, 2013. [pdf]
 |
|