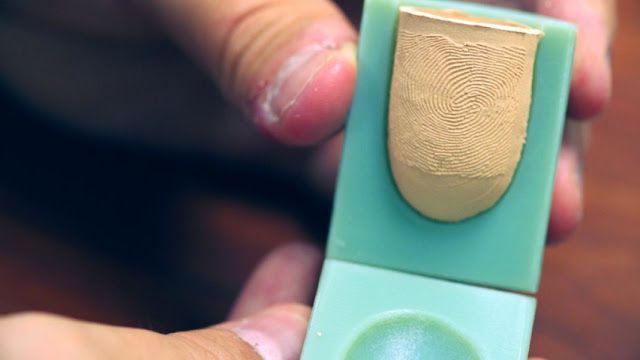
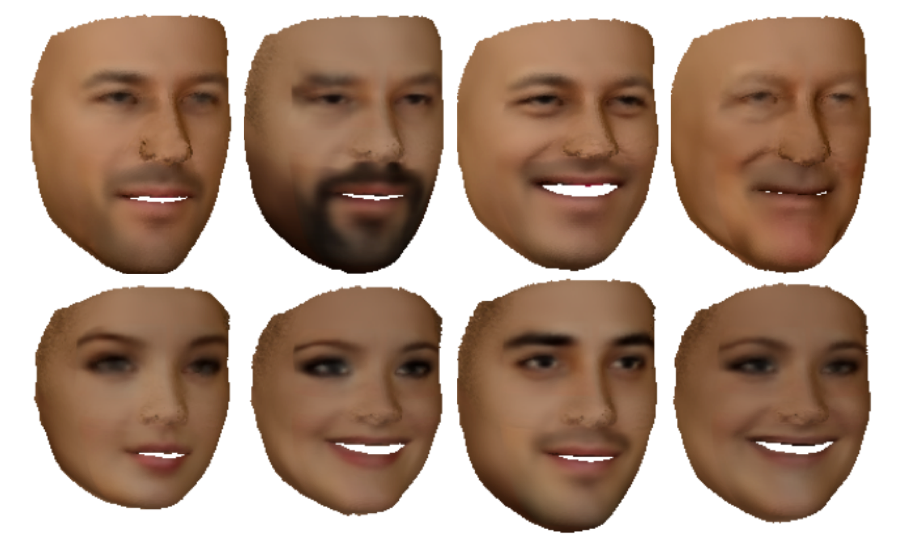
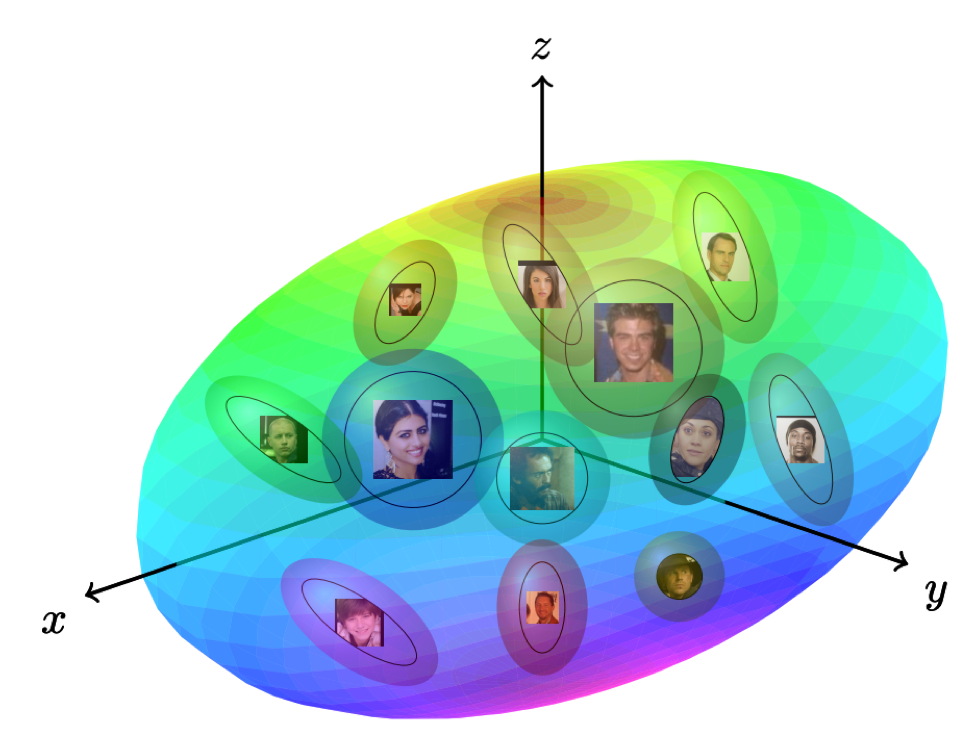
Location
Engineering Building, Rm 3540
Time
12:00 Noon - 1:00 PM
nformation
Lunch will be provided.
Upcoming Seminar: December 13, 2019
Face Phylogeny Tree: Deducing Relationships Between Near-Duplicate Face Images Using Legendre Polynomials and Radial Basis Functions
[BTAS 2019]
Presenter: Sudipta Banerjee (iPRoBe Lab)
Photometric transformations, such as brightness and contrast adjustment, can be applied to a face image repeatedly creating a set of near-duplicate images. These near-duplicates may be visually indiscernible. Identifying the original image from a set of near-duplicates, and deducing the relationship between them, is important in the context of digital image forensics. This is commonly done by generating an image phylogeny tree (IPT) - a hierarchical structure depicting the relationships between a set of near-duplicate images. In this work, we utilize three different families of basis functions to model pairwise relationships between near-duplicate images. The basis functions used in this work are orthogonal polynomials, wavelet basis functions and radial basis functions. We perform extensive experiments to assess the performance of the proposed method across three different modalities, namely, face, fingerprint and iris images; across different image phylogeny tree configurations; and across different types of photometric transformations. We also perform extensive analysis of each basis function with respect to their ability to model arbitrary transformations and to distinguish between the original and the transformed images. Finally, we utilize the concept of approximate von Neumann graph entropy to explain the success and failure cases of the proposed IPT reconstruction algorithm. Experiments indicate that the proposed algorithm generalizes well across different scenarios thereby suggesting the merits of using basis functions to model the relationship between photometrically modified images.
Location
Engineering Building, Rm 3105
Time
12:00 Noon - 1:00 PM
nformation
Lunch will be provided.
Seminar #2: November 8, 2019
Learning 3D Model from 2D In-the-wild Images [arXiv]
Presenter: Luan Tran (CV Lab)
Understanding the 3D world is one of computer vision's fundamental problems. While a human has no difficulty understanding the 3D structure of an object upon seeing its 2D image, such a 3D inferring task remains extremely challenging for computer vision systems. To better handle the ambiguity in this inverse problem, one must rely on additional prior assumptions such as constraining faces to lie in a restricted subspace from a 3D model. Conventional 3D models are learned from a set of 3D scans or computer-aided design models and represented by two sets of PCA basis functions. Due to the type and amount of training data, as well as, the linear bases, the representation power of these models can be limited. To address the aforementioned problems, we propose an innovative framework to learn a nonlinear 3D model from a large collection of in-the-wild images, without collecting 3D scans. Specifically, given an input image (of a face or an object), a network encoder estimates the projection, lighting, shape and albedo parameters. Two decoders serve as the nonlinear model to map from the shape and albedo parameters to the 3D shape and albedo, respectively. With the projection parameter, lighting, 3D shape, and albedo, a novel analytically-differentiable rendering layer is designed to reconstruct the original input. The entire network is end-to-end trainable with only weak supervision. We demonstrate the superior representation power of our models on different domains (face, generic objects), and their contribution to many other applications on monocular 3D reconstruction and analysis.
Location
International Center, CIP 115
Time
12:00 Noon - 1:30 PM
nformation
Lunch will be provided.
Seminar #1: October 11, 2019
Learning a Fixed-Length Fingerprint Representation [arXiv]
Presenter: Joshua J. Engelsma (PRIP Lab)
We present DeepPrint, a deep network, which learns to extract fixed-length fingerprint representations of only 200 bytes. DeepPrint incorporates fingerprint domain knowledge, including alignment and minutiae detection, into the deep network architecture to maximize the discriminative power of its representation. The compact, DeepPrint representation has several advantages over the prevailing variable length minutiae representation which (i) requires computationally expensive graph matching techniques, (ii) is difficult to secure using strong encryption schemes (e.g. homomorphic encryption), and (iii) has low discriminative power in poor quality fingerprints where minutiae extraction is unreliable. We benchmark DeepPrint against two top performing COTS SDKs (Verifinger and Innovatrics) from the NIST and FVC evaluations. Coupled with a re-ranking scheme, the DeepPrint rank-1 search accuracy on the NIST SD4 dataset against a gallery of 1.1 million fingerprints is comparable to the top COTS matcher, but it is significantly faster (DeepPrint: 98.80% in 0.3 seconds vs. COTS A: 98.85% in 27 seconds). To the best of our knowledge, the DeepPrint representation is the most compact and discriminative fixed-length fingerprint representation reported in the academic literature.